Ensure data-centricity to support AI and ML
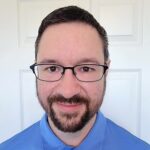
Andy Friedl
Principal Data Architect
Copy Link
Share on LinkedIn
Share on X
Share on Facebook
Share on WhatsApp